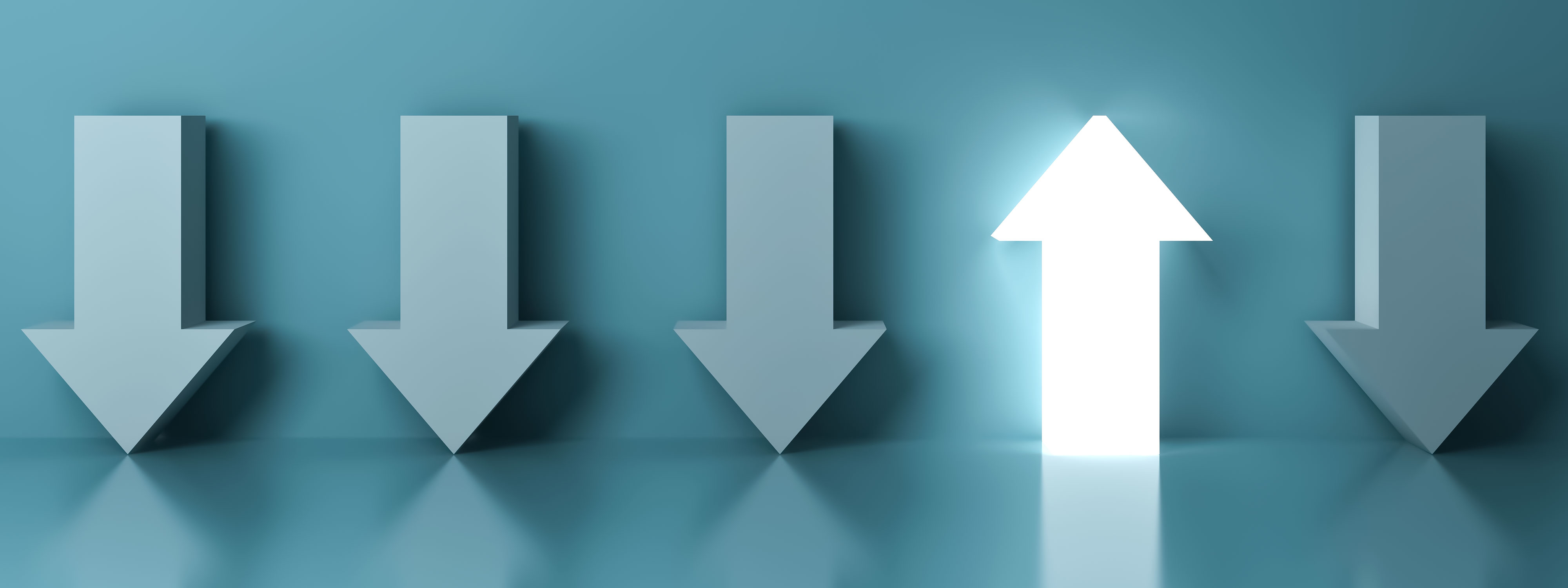
On 2 December 2019, the UK Information Commissioner's Office ("ICO") together with The Alan Turing Institute published1 a three-part consultation (with draft guidance) on explaining decisions made with Artificial Intelligence ("AI"), badged "Project ExplAIn". Part 1 is aimed at all staff involved in developing AI systems, including Compliance and the Data Protection Officer ("DPO"), and sets out the basics of explainability concepts. Part 2 sets out the practicalities of providing explanations of AI to individuals, and Part 3 focuses on the roles, policies, procedures and documentation necessary to ensure a business can provide meaningful explanations to affected individuals.
Background
The "Project ExplAIn" consultation was issued in response to the commitment in the UK Government's April 2018 AI Sector Deal to boost the UK's global position as a leader in developing AI technologies. This is not a statutory code of practice under the Data Protection Act 2018. Rather, the consultation comprises draft practical guidance on good practice for explaining data processing decisions made using AI systems to affected individuals. It clarifies the application of data protection law relevant to explaining AI decisions and identifies other relevant legal regimes outside the ICO's remit. Responses are due by 24 January 2020.
XAI Legal Framework
GDPR and DPA
The use of personal data to train, test or deploy an AI system falls within the scope of the General Data Protection Regulation ("GDPR") and the Data Protection Act 2018 ("DPA 2018"), which regulate the processing of personal data. Both the GDPR and the DPA 2018 include provisions specific to large scale automated processing of personal data, profiling and automated decision-making, and as such, directly govern the use of AI to provide a prediction or recommendation about individuals.
Any business seeking to comply with the GDPR and the DPA 2018, must be able to provide individuals with a meaningful explanation of its fully automated decisions that involve the processing of personal data. This facilitates the exercise of an individual's rights under data protection law, including the right of access, and the right to object and the right to obtain meaningful information, express their point of view, and contest fully automated decisions. Where a decision affecting an individual involves the processing of personal data and the use of AI (whether or not augmented with meaningful human involvement) the principles set out in the GDPR are relevant – especially those of fairness, transparency and accountability.
- Articles 13-14 of the GDPR require that businesses proactively provide individuals with "…meaningful information about the logic involved, as well as the significance and the envisaged consequences of such processing for the data subject…" in the case of solely automated decisions with a legal or similarly significant effect.
- Article 15 of the GDPR also gives individuals a right to obtain this information at any time on request.
Even where decision making systems are not fully automated, and there is a "human in the loop", the ICO recommends that these principles should be followed as good practice.
Equality Act and Administrative law
The Equality Act 2010 applies to those businesses using AI in their decision-making process, who must show that this does not result in discrimination. Judicial review under administrative law is also available as a means of challenging decisions made by the public sector or by private sector entities contracted by government to carry out public functions which deploy AI to support decision-making, on the basis that the decision was illegal or irrational, or the way in which it was made was "improper".
XAI Explanation types and Principles
The ICO identifies six primary explanation types:
- Rationale explanation: the reasons that led to a decision, delivered in an accessible and non-technical way, which also assists businesses with GDPR compliance;
- Responsibility explanation: who is involved in the development, management and implementation of an AI system, and who to contact for a human review of a decision;
- Data explanation: what data have been used in a particular decision and how; what data have been used to train and test the AI model and how;
- Fairness explanation: steps taken across the design and implementation of an AI system to ensure that the decisions it supports are generally unbiased and fair, and whether or not an individual has been treated equitably;
- Safety and performance explanation: steps taken across the design and implementation of an AI system to maximise the accuracy, reliability, security and robustness of its decisions and behaviours; and
- Impact explanation: the impact that the use of an AI system and its decisions has or may have on an individual, and on wider society.
The ICO also specifies four principles which businesses should follow:
- Be transparent: render the technical rationale underlying the model's output comprehensible and give plain-language reasons which affected individuals can easily evaluate;
- Be accountable: consider accountability at each stage of the AI system's design and deployment and whether design and implementation processes been made traceable and auditable across the entire project;
- Consider the context in which the business operates; and
- Reflect on the impact of the business's AI on the individuals affected, as well as on wider society, e.g. whether the model has been designed, verified and validated to ensure it is secure, accurate, reliable and robust.
XAI Organisational Functions and Policies
The ICO requires all those involved in a business's decision-making pipeline to participate in providing an explanation of a decision supported by an AI model's result. Under data protection law, controllers are responsible for ensuring that any AI system whose development is outsourced is explainable.
Key areas to cover in businesses' policies and procedures are set out, including documenting both the processes behind the design and implementation of the AI system and the actual explanation of its outcome.
Where opaque algorithmic techniques, whose inner workings and rationale are inaccessible to human understanding (such as black box AI) are used, businesses must identify the ancillary risks, show how these risks are mitigated via supplementary explanatory algorithms or other tools, and provide evidence of any determination that their use case and organisational capacities support the system's responsible design and implementation. Businesses must also show that any potential for biases in the model design are assessed, monitored and mitigated.
XAI in practice
The ICO's draft guidance sets out the practicalities of explaining AI-assisted decisions and providing explanations to individuals, including how to choose a sector and use case-appropriate explanations and tools for extracting explanations from less interpretable models.
The ICO also provides technical teams with a comprehensive guide to choosing appropriately interpretable models and supplementary tools to render opaque black box AI determinations explainable. Further resources for exploring XAI are listed in the Appendix to the draft guidance.
The ICO's suggested steps include the following:
- Select priority explanations by considering the domain, use case and impact on the individual;
- Collect the information required for each explanation type;
- Build a rationale explanation to provide meaningful information about the AI system's underlying logic;
- Translate the rationale of the AI system's results into usable and easily understandable reasons;
- Prepare implementers to deploy the AI system;
- Consider contextual factors when delivering the explanation; and
- Consider how to present the explanation.
The draft guidance divides each type of explanation into two categories:
- Process-based explanations of AI systems, which show that good governance processes and best practices have been followed throughout design and use;
- Outcome-based explanations of AI systems, which clarify the results of a particular algorithmically generated result in plain language. Affected individuals must also be shown how and why a human judgment augmented by AI output was reached.
Appropriately explainable models should factor-in cases where social or demographic data are being processed, which may involve particular issues of bias and discrimination, and thus drive the choice of an optimally interpretable model, avoiding black box AI. These techniques may include decision trees or rule lists, linear regression and generalised additive models, case-based reasoning, or logistic regression.
Opaque black box AI and supplementary algorithms which can explain it
The ICO warns that black box AI systems, including neural networks and random forests, should only be used where their potential impacts and risks have been thoroughly considered. A business's use case and organisational capacities must support the responsible design and implementation of black box AI, and any supplemental interpretability tools should provide the system with sufficient explainability to mitigate the identified risks and give affected decision recipients meaningful information about the rationale of any outcome.
Helpfully, the ICO sets out a detailed list of the supplementary techniques which can provide some access to the underlying logic of black box AI models. The ICO's research has shown that, while the banking and insurance sectors are continuing to select interpretable models in their customer-facing AI decision-support applications, they are increasingly using more opaque black box "challenger" models alongside these for the purposes of benchmarking, comparison and insight. The ICO notes that, where challenger models are used to process the personal data of affected decision recipients even for benchmarking purposes, they should be properly recorded and documented.
The ICO guidance includes a table listing the basic properties, potential uses and interpretability characteristics of the currently most widely used algorithms, of which, the first 11 are considered to be mostly interpretable, and the final four to be primarily black box algorithms. The AI systems listed by the ICO mostly produce statistical outputs based on correlation rather than causation, so businesses are warned to sensecheck whether the correlations produced by the AI model make sense for each relevant use case.
Peeking into the black box: local vs global and intrinsic vs external explanations
The draft guidance draws a distinction between the explanation of single instances of an AI model's results (a "local explanation" aiming to interpret individual predictions or classifications) and that of how it works across all its outputs (a "global explanation" capturing the inner logic of that model's behaviour across predictions or classifications).
The ICO also notes that applying an internal or model-intrinsic explanation to black box AI can shed light on that opaque model's operation by breaking it down into more understandable, analysable and digestible parts. For example, in the case of an artificial neural network (ANN) it can break it down into interpretable characteristics of its vectors, features, interactions, layers and parameters (often referred to as "peeking into the black box").
By contrast, external or post-hoc explanations are more applicable to black box systems where it is not possible to access fully the internal underlying rationale due to the model's complexity. These attempt to capture essential attributes of the observable behaviour of a black box system by reverse-engineering explanatory insights.
Sense-check the statistical rationale
Understanding the correlations or statistical rationale between input variables and an AI model's result is described in the draft guidance as the first step in moving from the model's mathematical inferences to a meaningful explanation. But, as the ICO notes, "correlation does not imply causation" and the guidance requires additional steps to assess the role that these statistical associations should play in a reasonable explanation, given the specific context.
Train humans to deploy AI non-anthropomorphically
Where decisions are human-augmented rather than fully automated, human implementers must be appropriately trained to use the model's results responsibly and fairly, including on the basics of how machine learning works, the limitations of AI and automated decision-support technologies and the benefits and risks of deploying these systems (especially how they augment, rather than replace, human judgment). Training must highlight the risks of cognitive biases, such as overconfidence in a prediction based on the historical consistency of data, or illusions that any clustering of data points necessarily indicates significant insights. The ICO warns that any training should avoid anthropomorphic portrayals of AI systems.
Where decisions are fully automated and provide a result directly to the decision recipient, businesses should set up the AI system to provide understandable explanations.
What does this mean for market participants?
The ICO warns that businesses that do not explain AI-assisted decisions could face regulatory action, reputational damage and disengagement by the public. The ICO notes that process-based and outcomebased explanations relating to the rationale of an AI system, and an outcome-based explanation relating to the AI system's impact on the individual, can fulfil the requirements in Articles 13-15 of the GDPR – but that it is up to each business to determine the most appropriate way to deliver the explanations which that business elects to provide.
The regulator is also aware, however, that businesses may need to protect against the risk of third parties gaming an AI model if they know too much about the reasons underlying its decisions. It is therefore essential for businesses that are using AI for data processing purposes to find an appropriate balance that provides individuals with an explanation of how their data will be processed, without exposing the AI systems to the risk of attack by malicious third parties.
Click here to download 'XAI: Explainable AI – Data Protection Hobbling the Prediction Machine?' PDF
1 https://ico.org.uk/about-the-ico/ico-and-stakeholder-consultations/ico-and-the-turing-consultation-on-explaining-ai-decisions-guidance/ The UK Financial Conduct Authority (FCA) is also working with The Alan Turing Institute on AI explainability for financial services firms, having highlighted the explainability of decisions made on the basis of black box algorithms and data privacy in its April 2019 Research Agenda at https://www.fca.org.uk/publication/corporate/fca-research-agenda.pdf. An FCA speech this summer at https://www.fca.org.uk/news/speeches/future-regulation-ai-consumer-good noted that the FCA and the Institute will be undertaking a joint publication on AI explainability, with a workshop planned for early 2020.
This publication is provided for your convenience and does not constitute legal advice. This publication is protected by copyright.
© 2019 White & Case LLP